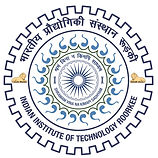
BROAD RESEARCH DOMAIN
Our research focussed on the thematic area of electronic design automation (EDA). EDA refers to the development of software tools required for the behaviroal modeling, analysis, virtual prorotyping, and performance assessment of electronic devices and circuits. These tools are of very high value to circuit designers because they can be used to precisely determine how the performance of a device/circuit can be tuned for optimal perfromance without the need of costly and labor-intesnive fabrications, design runs, characterizations, and experimental measurements.
​
Some of the critical areas where the CMAS laboratory is currently working involves:
-
Uncertainty quantification and stochastic modeling of high speed devices and ircuits
-
Signal and power integrity verification in emerging interconnects
-
Machine learning for fast design space exploration of nanoscale FET devices
-
CAD of spintronic devices for non volatile memories and radiation-hardened circuits
-
Variability analysis of silicon-photonic components, devices, and photonic ICs
-
Modeling of memrisitive circuits for nueromorphic computing applications
​
​
​
Funding Sources:
Ministry of Human Resource Development, Govt. of India,
Faculty Initiation Grant IIT Roorkee,
Startup Research Grant, SERB, Govt. Of India
Uncertainty quantification entails mathematically predicting the impact of probabilistic and non-probabilistic variability in the input parameters of a device or circuit on the output responses of interest. The variability in the input parameters arises from the fabrication process variations, manufacturing tolerances, and unpredictable operating conditions. Currently, the state-of-the-art uses surrogate models/metamodels to perform uncertainty quantification.
​
Within this topic area, the key questions our research is interested in are the following.
(i) How do we developing fast algorithms to efficiently train the surrogate models/metamodels in the presence of high-dimensional uncertainty?
(ii) How do we make the training algorithms flexible enough to include the effects of both probabilistic and non-probabilistic uncertainty?
(iii) How do we develop fast training algorithms where very sparse data regarding the input variability is available?
(iv) How do we improve the convergence of the metamodel to the true results?
​
More recently, our research group is involved in exploring the use of machine learning (ML) based regression models for infering the statistics of device and circuit responses in the presence of high-dimensional uncertainty. It is our expectation that ML based models can mimic the highly nonlinear I/O relationships in very high-speed circuits.
​
​
​
​
​
​
Funding Sources:
Ministry of Human Resource Development, Govt. of India,
Faculty Initiation Grant IIT Roorkee,
Startup Research Grant, SERB, Govt. Of India
​
As technology nodes scale down to the sub-45 nm levels, the decreasing cross-sectional area of conventional copper interconnects lead to increased grain boundary and side-wall scattering effects. This, in turn, translates to higher interconnect resistance and consequently, larger RC signal delay, greater signal attenuation, increased power loss, and increased chances of thermal breakdown and electromigration. Thus, new and emerging interconnects based on graphene (e.g., single-walled carbon nanotube (SWCNT), multi-walled carbon nanotube (MWCNT), and multilayer graphene nanoribbon (MLGNR) networks) are emerging as promising alternatives to copper interconnects. Besides pure graphene based interconnects, composites such as copper-graphene hybrid interconencts are also currently of high interest.
​
​
​
​
​
​
​
The critical modeling challenges our research seeks to answer regarding these graphene-based interconnect technologies are the following.
(i) How do we account for the quantum effects inside the SPICE model of such interconnects?
(ii) How can we perform fast deterministic and stochastic analysis of such interconnects given that the MWCT and MLGNR networks are massively large and coupled?
(iii) How can we quantify the impact of self-heating on the performance of these interconnects?
(iv) Given the highly complex and cumbersome multi-shell and multi-conductor structure of graphene based interconnects, how can SPICE simulations of such networks be done efficiently?
​
​
​
​
​
​
Funding Sources:
Ministry of Human Resource Development, Govt. of India,
Qualcomm Innovation Fellowship India 2021
With the sustained miniaturization of transistir feature sizes to below the 22 nanometer mark, bulk CMOS devices can no longer meet the power, performance and are (PPA) requirements. Hence, more sophisticated FET devices such as FinFET, negative capacitance FET (NC-FET), and nanosheet FETs are emerging as replacements of bulk CMOS devices. These devices have many input geometrical, material, and electrical parameters that can be tuned to optimize the circuit level performance. IN order to ensure fast design optimization, uncertainty quantification, and worst-case analysis, closed-form but accurate I?O models of these devices are required. Our research team is exploring how ML based regression models can fill this gap.
​
​
​
​
​
​
​
​
​
The critical modeling challenges that we are attempting to asnwer in this field are the following.
(i) How do we develop physics-based equivalent SPICE circuit models for these devices?
(ii) How can we reliably perform the small signal analysis of such FET devices, especially in the millimeter frequency ranges without resorting to highly expensive numerical TCAD simualtions?
(iii) When using ML based regresion models for large-signal or small-signal analysis, how can we address the need for massive amounts of training data?
(iv) For nanoscale FETs, how can we speed up the computational cost of multiphysics simualtions such as electro-thermal simualtion for studying self-heating effects?
​
​
UNCERTAINTY QUANTIFICATION AND STOCHASTIC MODELING
SIGNAL AND POWER INTEGRITY VERIFICATION IN EMERGING INTERCONNECTS
MACHINE LEARNING FOR FAST DESIGN SPACE EXPLORATION OF NANOSCALE FET DEVICES
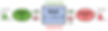
Source: Jiaxin Zhang*. "Modern Monte Carlo methods for efficient uncertainty quantification and propagation: A survey". WIREs Computational Statistics, 2020
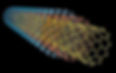

Source: R. Karimi, M. Moaiyeri, S. G. Hamedani, "An ultra‑energy‑efcient crosstalk‑immune interconnect architecture based on multilayer graphene nanoribbons for deep‑nanometer technologies," Journal of Computational Electronics (2021)


Source: S. M. Goodnick, A. Korkin, and R. Nemanich, Semiconductor Technology, Springer 2018